Intelligent Data Integration Uses the Coordinates
Spatial Components Improve Results
Only valid and consistent data provide added value. Automated data integration saves resources. A new development from WIGeoGIS helps. We use the spatial reference that is included in most data, thereby improving the results.
Data, the “Gold of the 21st Century”?
Has this happened to you before? – You have five different spreadsheets with valuable data and you want to continue working with them all. But it turns out they are not compatible. The spreadsheets have different columns, the formats are different, duplicates falsify your results, but are not so easy to recognize and delete.
You are not alone with these problems!
In practice, such tasks usually require extensive manual effort. And just like that, the much-praised “gold of the 21st century” is not as shiny anymore and you can not raise your treasure.
Practical example: All customers minus existing customers = potential new customers
It sounds simple: You would like to determine your potential new customers using the dataset of a commercial provider (e.g. yellow pages). The data of your existing customers are available in your own CRM database. All you have to do is filter those existing customers out of the yellow pages and you will know who your potential new customers are.
Those who have never dealt with something like this are sure to think it is a simple task that can be done with the “touch of a button”!
Difficulty integrating location data? Tell us your problem. We are the specialists!
The Challenge of Data Integration
Preparing data from different sources for evaluation together is called data integration. Most of the time the data is redundant and inconsistent. Another example: You want to create a complete, valid list of all the hotels in a region using different spreadsheets from your own data, local yellow pages, government data, data from internet research.
Many of the hotels are in several or all of the lists, so you have to merge the duplicates. Presumably, each record will contain a name. But beware, some hotels will appear under different names, e.g. due to ownership changes. Only by comparing the address data, will you be able to clean up the lists.
Furthermore, some hotels will appear under slightly different coordinates, even though they are really at the same location. How do you find these duplicates? And if a location exists as a point in one dataset and as a polygon in another dataset, how are the two related, and how do you display it?
Presumably, you will also be struggling with the different formats that have been established for location data over the years. The available metadata is different. One spreadsheet contains the number of beds, in another one this data is missing, but there are entries about the facilities and price.
Until you have one consistent spreadsheet that you can actually work with, you will need to invest a lot of time and effort!
SLIPO Helps for Data with a Spatial Reference
As part of an EU-funded research project called SLIPO, WIGeoGIS worked together with partners from research and the industry to develop a tool and method set with which we are pursuing exactly this goal: this one consistent table that is largely automated, faster and more efficient.
How does it work? – We use the spatial component included in the data!
Because nearly 80% of all company data has a spatial reference.
Not just classic location data (such as branches and other real estate), but also your customer data, which of course includes an address. If the coordinates are the same when integrating data from different sources, the systematic, automated evaluation of the remaining attributes provides the information as to whether they are the same content/data set. This not only saves time, but also results in better data.
The illustration clearly shows the process of integration, validation and quality-assured enrichment of location data or data that have a coordinate (“Point of Interest” data). Behind this process lie the innovative and complex so-called linked-data technologies.
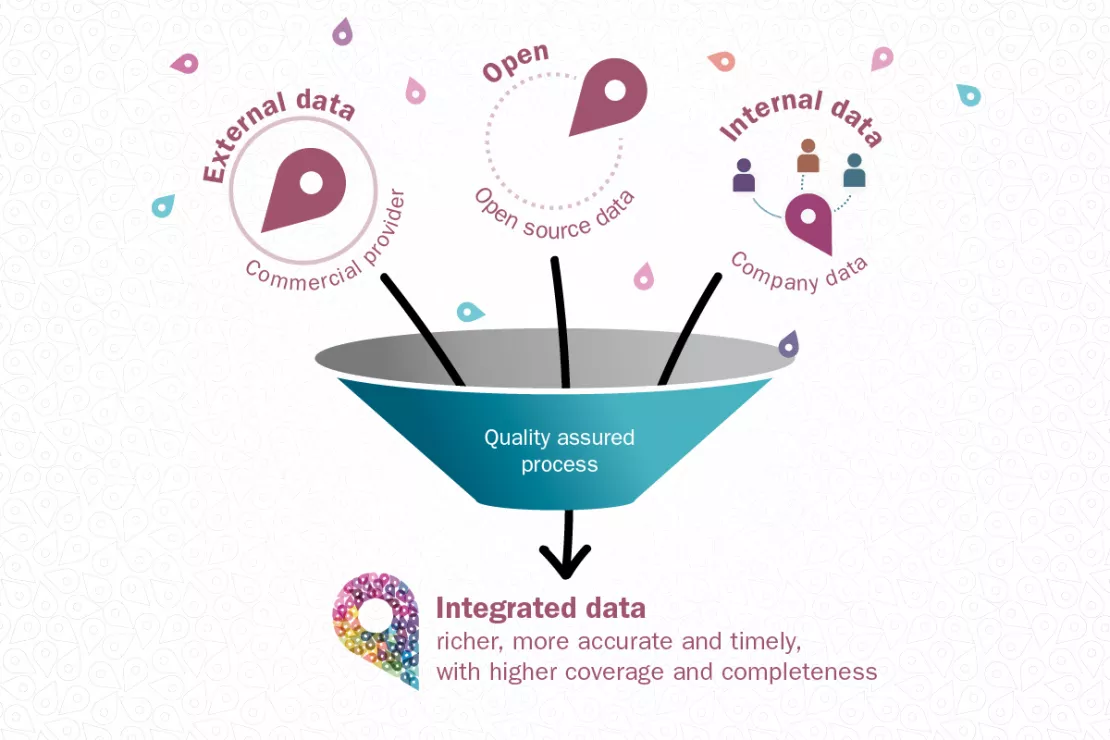
You have location data from different sources and want to get the most out of it with as little effort as possible? - We can help you!
Test Case Munich Re
In cooperation with Munich Re, we subjected our development to a practical test.
Munich Re is a reinsurer, in other words it insures insurance companies (“primary insurers”). The company is globally active and is one of the largest in its sector. Assessing global locations for risk is one of their core responsibilities.
For them, location data that is complete and geographically accurate is essential. In addition to their own location databases (e.g. natural hazard maps), Munich Re uses a large number of different data sources from both commercial and OpenData location data providers. Currently, merging data sources at Munich Re requires extensive manual effort.
In the test, address lists of hotels from four different sources were merged or integrated. The problems were similar to those described above.
Success!
Our tools have proven themselves and are ready for further projects.
Applied Research Brings Results
“For me, this partnership is a promising example of how goal- and practice-oriented research and business have to work together to be successful.”
Andreas Siebert, Head of Geospatial Solutions, Munich Re
Intelligent Data Integration: Request a free initial consultation
- FREE initial consultation on Intelligent Data Integration
- Non-binding, without further obligations
- By telephone or video call
Do you want to integrate your data intelligently? I will gladly support you. Please contact me!